Category: Artificial Intelligence News
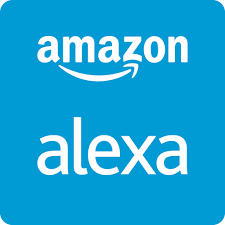
Amazon Again Topped Q4 Global Smart Speaker Sales Followed by Google and Baidu According to Strategy Analytics. Smart Speaker Shipments Set New Record.
Mar 15, 2020
Amazon shipped 15.8 million smart speakers in Q4 2019 capturing a 28.3% device market share and the top spot globally Google followed Amazon with a…
Read More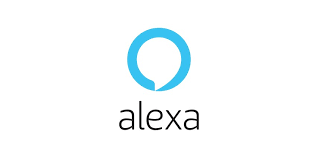
U.S. In-car Voice Assistant Users Rise 13.7% to Nearly 130 Million, Have Significantly Higher Consumer Reach Than Smart Speakers – New Report
Mar 15, 2020
Total in-car voice assistant users in the U.S. is almost 130 million with 83.8 million active monthly This total reflects a 13.7% rise in users…
Read More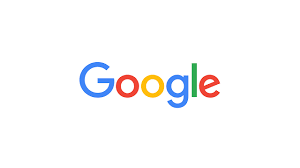
Over 60% of Car Buyers That Have Used Voice Assistants Factor Availability into Purchase Decision
Mar 15, 2020
Over three-fifths of consumers that have used a voice assistant while driving say they factor in assistant availability when considering a new car purchase. This…
Read More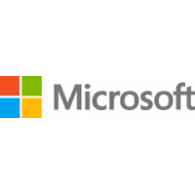
Microsoft’s Earbuds Put the Office 365 in Your Ears
Mar 15, 2020
MICROSOFT AUDIO OFFICE The Surface Earbuds offer a variation on the usual features of wireless earbuds. Each rests inside the ear behind a sizeable white…
Read More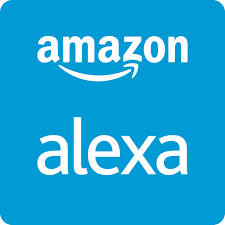
Amazon’s roadmap for Alexa is scarier than anything Facebook or Twitter is doing
Nov 11, 2019
Amazon has big plans for its virtual assistant. One day, perhaps sooner than you think, Alexa will take a proactive role in directing our lives.…
Read More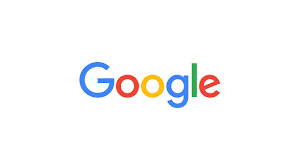
Google: Follow Our Structured Data Requirements to Ensure Rich Result Eligibility
Oct 10, 2019
Google’s John Mueller recommends following the company’s official structured data requirements to ensure content is eligible to be displayed as a rich result. This topic…
Read More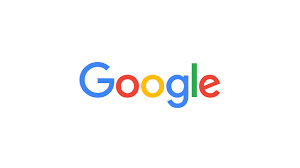
Google is Testing Search Results Without URLs – We may be looking at the beginning of the end for URLs in search results.
Oct 08, 2019
Google appears to be testing the complete removal of URLs from search results, displaying only the website name instead. This was spotted by a Reddit…
Read More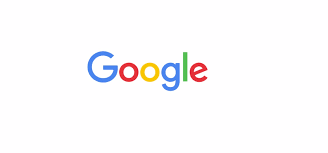
Google Search: Ongoing changes to the SERP design, directing more traffic towards paid advertisements
Oct 04, 2019
That means over half of Google’s search engine results lead to no clicks through to websites or properties that aren’t owned by Google. This data was…
Read More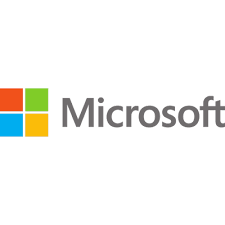
Adobe, Microsoft and LinkedIn Join Forces to Accelerate Account-Based Experiences
Sep 25, 2019
Adobe (Nasdaq:ADBE) today announced an extension of its partnership with Microsoft and a new integration with LinkedIn that will accelerate account-based experiences (ABX) through new…
Read More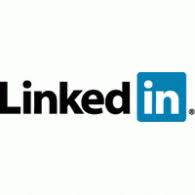
LinkedIn Announces New Data Partnership with Adobe to Improve Ad Targeting
Sep 25, 2019
LinkedIn continues to expand its audience targeting capability, this time through a new partnership with Adobe which will expand LinkedIn's account-based marketing capabilities to Adobe…
Read More