Category: Artificial Intelligence News
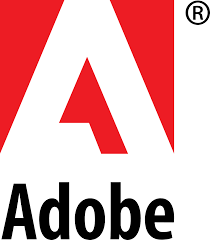
Adobe Expands Integrations With AI-Drive Account Profiling, LinkedIn Synching
Sep 21, 2019
Adobe has launched new capabilities within the Marketo Engage ABM Essentials offering, part of the Adobe Marketing Cloud, including new AI-powered models in its Account Profiling…
Read More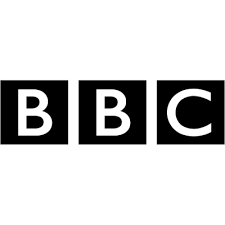
NLG in the newsroom: fast, consistent, and hyperlocal
Aug 26, 2019
NLG in the newsroom: fast, consistent, and hyperlocal We’ve written about how Natural Language Generation can eliminate the bottleneck of manual, one-at-a-time analysis within business…
Read More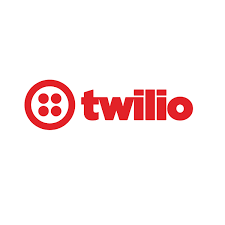
Twilio: Harnessing The Power Of AI (Artificial Intelligence)
Aug 26, 2019
Twilio, a provider of voice, video and messaging services, reported its second quarter results and they were certainly standout. Revenues spiked by 86% to $275…
Read More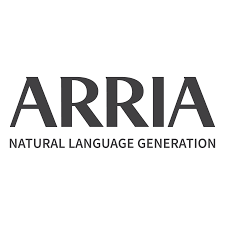
Artificial Intelligence Search, NLP & Automation
Aug 26, 2019
Another significant requirement is the need to find an efficient method for reducing the amount of computational searching for a match or a solution. Considerable…
Read More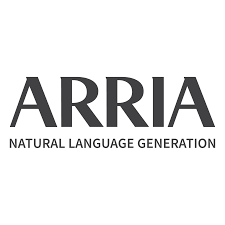
Amazon’s Alexa as a presentation layer over a Tableau dashboard integrated with Arria’s NLG technology
Aug 20, 2019
A Big Day for Arria at VOICE Summit 2019 Greg Williams I write this dispatch while sitting with Arria teammates outside of the crowded meeting…
Read More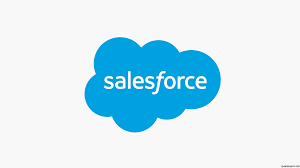
Analytics Are Defining The Future Of Digital Advertising
Aug 19, 2019
Advertisers are planning to increase the average number of integrated data sources from 5.4 today to 6.2 in 2019, searching for new customer and advertising…
Read More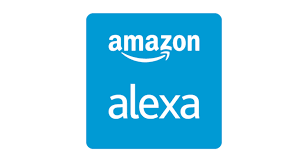
Healthcare Virtual Assistants Market Expected to reach $3,133.6 million by 2026 growing at a CAGR of 32.7%
Aug 10, 2019
Global Healthcare Virtual Assistants Market is accounted for $245.7 million in 2017 and is expected to reach $3,133.6 million by 2026 growing at a CAGR…
Read More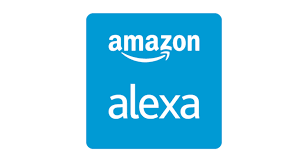
Amazon brings Alexa announcements to Fire TV and completes YouTube rollout
Aug 10, 2019
Amazon today announced that its Alexa announcements feature is launching on all Fire TV devices in countries where the intercom-like functionality is available. It’s useful…
Read More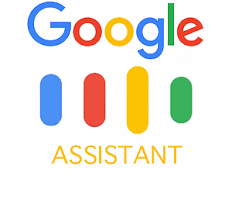
Google makes a subtle but important change to the Search app
Jul 29, 2019
A look at the updated version of the Google Search app reveals that Google is phasing out its Voice Search feature in favor of the Google…
Read More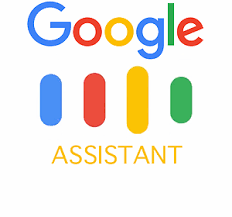
Google Assistant has started to take charge of ‘Voice search’ in Google app
Jul 29, 2019
The ability to search the web with the voice came way earlier than digital assistants. And now with digital assistants, users are able to not…
Read More